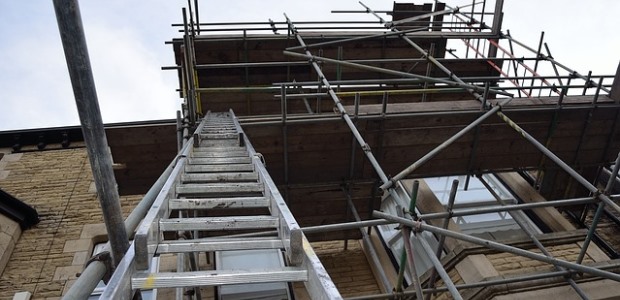
Three Reasons Why Construction Companies Fail When Trying to Predict Injuries
We need to change the mindset these machine-based methodologies must be viewed skeptically or cynically.
- By Griffin Schultz
- Aug 01, 2014
A study in collaboration with a group of Carnegie Mellon University researchers found that future workplace injuries can be predicted with accuracy rates as high as 80-97 percent. By applying these predictive analytics practices in the real world, construction companies are successfully predicting and preventing workplace injuries, resulting in millions of dollars in savings, stronger safety cultures, and increased workplace productivity.
Hard to believe? Why? Predictive analytics has been used successfully for years in other business functions, such as sales, marketing, and finance. A recent study by Ventana Research claims that predictive analytics has "entered the mainstream." Bain and Company found that companies who adopt "big data" analytics are twice as likely to be in the top quartile of financial performance within their industries.
Predictive analytics works, and now it's being used to save lives on construction job sites around the globe. Nonetheless, when contractors try to implement predictive analytics, they often struggle with three main challenges.
Challenge #1 – The Wrong Tool for the Job
A helicopter is a very powerful and versatile tool. However, you could never get to the moon with one. Some pretty basic laws of physics simply make it impossible. The same is true when attempting to predict workplace safety incidents using Microsoft Excel or other, similar tools. Some simple laws of math and computational power are going to hold you back.
In his book "Competing on Analytics," Tom Davenport suggested that basic data analytics tools allow us to answer only simple business questions such as "what happened, how many, how often, and where." These sound much like the lagging indicators that most construction safety professionals are trying to shed nowadays. Lagging indicators can tell us only what has already happened. While such information is readily available in basic data tools that allow us to query data and run reports, these basic activities don’t transform data into actionable information. One of the best quotes I ever heard is, "Leaders don't want reports, they want answers."
In order to get the most out of our data and answer more strategic business questions such as "why is this happening" and "what if these trends continue," Davenport suggests that we use more advanced analytics capabilities, such as statistical analysis, forecasting, and extrapolation. Ultimately, he contends, if we want to predict the future by answering the question of "what will happen next," we need to employ predictive analytics. This simply can't be done effectively with basic analytics tools.
Well beyond Excel, many of the most cutting-edge advances in predictive analytics are coming from the field of machine learning. Machine learning, one of the highest forms of predictive analytics, is when computers learn without being explicitly programmed by humans. They learn simply by consuming extremely large data sets. Using advanced analytics techniques such as support vector machines, decision trees and forests, and neural networks, computers can unlock trends, patterns, and correlations in the data in a way that humans and basic analytics tools cannot.
If you want to go to the moon, you're going to need a rocket ship. If you want to predict workplace injuries, you're going to need more powerful analysis tools than Excel.
Challenge #2 -- Not Enough 'Big Data'
Once you have a rocket ship, you're going to need to fill it with a very powerful fuel in order to get to the moon. If you’re going to employ advanced analytics tools, predictive models, and even machine learning algorithms, you're going to need to fuel them with a very big data set. Machine learning prediction techniques can be extremely accurate and effective, but the machine needs a vast amount of data in order to learn.
In today's era of big data, this seems easy. After all, according to IBM, 2.5 quintillion bytes of data are created every day--that's the number 25 with 18 zeroes after it. IBM also claims that 90 percent of the world's data has been created in the last two years; 2.9 million emails are sent every second, 20 hours of video is uploaded to YouTube every minute, and Google processes 24 petabytes of data per day.
Construction companies specifically are collecting more and more safety data every day in the form of job safety analyses, inspections, audits, observations, near misses, root causes, and contributing factors. But except for the biggest of the big general contractors (GCs) who manage hundreds of projects a year, it takes most contractors several years to amass a big data set sufficient to drive high-powered machine learning programs.
Even if contractors, including large GCs, are able to amass enough independent variables (those used to predict safety incidents), they might not have enough historic dependent variables (those things we are trying to predict; in this case, safety incidents). If you lack enough of either variable type, the machines can't learn. Further, if you have just enough to train the machines, you could be without enough data to test the predictive algorithms that the machine has developed. Regardless, you need a big data set that is often beyond the reach of most contractors on their own.
To overcome this challenge, construction companies often need to pool their data sets with other contractors whose data is structured like theirs. This data pooling is what the aforementioned Carnegie Mellon team used to drive their predictive analytics results.
Challenge #3 – People Don't Trust Machines
In many ways, humans simply have a hard time trusting machines. This is changing over time as people become more accustomed to interacting with machines in everyday life, but hesitation still lingers.
Some of us are skeptical as to what machines can actually accomplish. Take me, for example, as a relatively tech-savvy professional who is old enough to have relied on paper maps for many years. I am still skeptical that Siri is going to get to me to my destination without getting me lost. The same is true for most people in regard to predictions that come from machines. There are many folks who simply do not believe that machine-based prediction methodologies are valid or reliable. They believe that humans are better predictors, especially in the field of construction safety, where human behavior and ever-changing job sites play such strong roles in safety outcomes.
However, research shows that we should have a little more faith. In a blog post titled "Are You Smarter than an Algorithm?" based on some of famed researcher Daniel Kahneman's work, Jim Harris suggested that "humans are incorrigibly inconsistent in making summary judgments of complex information. When asked to evaluate the same information twice, they frequently give different answers." Harris went on to say that "Kahneman' s review of separate studies on the reliability of judgments made by auditors, pathologists, psychologists, organizational managers and other professionals revealed that they contradicted themselves 20 percent of the time when asked to evaluate the same case on separate occasions." Kahneman was quoted as saying that, "by contrast, algorithms do not suffer from such problems. Given the same input, they always return the same answer."
In a Harvard Business Review blog post about “Convincing People NOT to Trust Their Judgment,” Andrew McAfee came to similar conclusions. According to McAfee, “as the amount of data goes up, the importance of human judgment should go down. Human intuition is real but it’s also really faulty.” McAfee cited several examples in which humans did worse than machines in predicting outcomes:
- Human parole boards do much worse than simple formulas at determining which prisoners should be let back on the streets.
- Highly trained pathologists don’t do as good a job as image analysis software at diagnosing breast cancer.
- Purchasing professionals do worse than a straightforward algorithm predicting which suppliers will perform well.
Further, some of us go in the opposite direction and are cynical about machines simply because of how powerful they have become. Is it truly irrational to think that Siri may “close the pod bay doors” on me just as HAL did to astronaut David Bowman in the movie “2001: A Space Odyssey”? I am not so sure.
Regardless, I have encountered professionals in construction safety who are concerned about computers taking over their job. As someone who works in this field every day, I can tell you authoritatively that this is not going to happen any time soon. For a real-world example of how computers and machines can aid construction safety professionals in their pursuit of zero incidents, simply watch the videos on YouTube of how the super-computer Watson, which beat the top human contestants on the game show Jeopardy, is helping doctors–but not replacing them–in making complex medical diagnoses.
While these three challenges to prediction are real, we can’t let them stop construction companies from employing these cutting-edge strategies in their safety programs. As Bain and others have found, those that do perform better and achieve better outcomes.
Generally through technology innovation, we must ensure that analytics tools are accessible and easy to use so that construction safety professionals can make a difference with their use. We must also demand access to the big data sets that will serve as fuel for these analytic tools, whether they reside in government agencies, think tanks, or even construction industry trade groups. Finally, we need to change the mindset that these machine-based methodologies need to be viewed skeptically or cynically. After all, the lives of those we are trying to protect are at stake.
This article originally appeared in the August 2014 issue of Occupational Health & Safety.