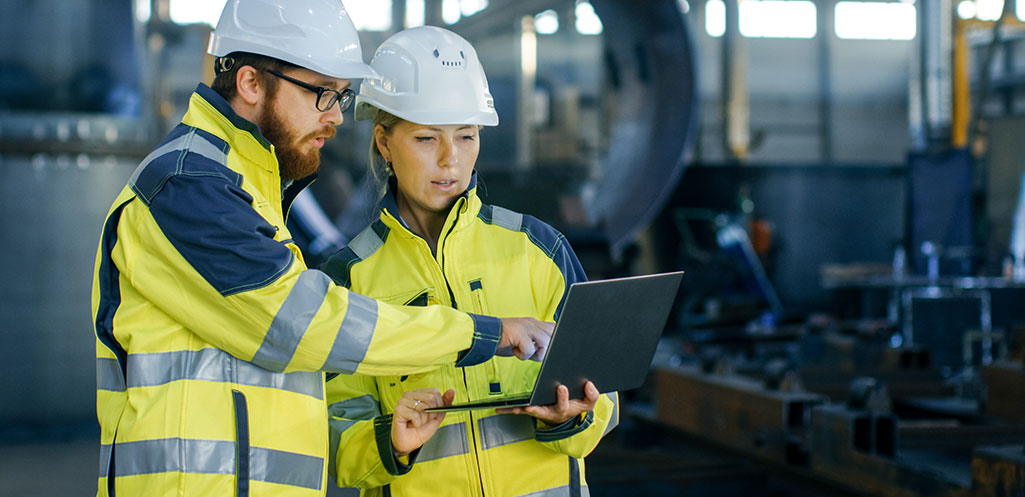
When Human and Machine-Generated Data Come Together: The Future Approach to Advancing Worker Safety
- By Pamala Bobbitt
- Feb 25, 2018
Safety managers are making an impact. While accident rates have fallen from a rate of 4,000 per 100,000 workers in 2000 to half that figure in 2015, these rates are still too high and the damages associated with them are still too extreme. As organizations look to continuously improve workplace safety, EHS departments are now delving deeper into their data to better understand why incidents occur. Yet, frustrations remain as safety professionals express they don’t have the time or know-how to properly analyze their data. To help with this, software and IoT vendors are leveraging amassed data to create algorithms that identify personnel, equipment, and other resources at risk of injury or incident. These point solutions will help to decrease accident rates, however, it won’t be until human-captured data is combined with machine-generated data that we’ll truly have a holistic view into workplace safety to drive change.
Plan for Tomorrow, Today
Organizations are steadily realizing and investing in technologies to support EHSQ initiatives. According to the 2017 Verdantix Green Quadrant, over 46% of respondents had at least a wearable safety device pilot program – if not a rollout – across all relevant locations and 41% had some type of EHS mobile app deployment in 2017. When you combine these initiatives with plans for IIoT and Industry 4.0 adoption, the volume of available data and opportunity for increasing workplace safety will exponentially grow in tandem.
This change is resulting in an immediate shifting of resources to better capture, cleanse, and analyze EHS data to drive safer outcomes.
Data Capture
With global adoption of EHSQ software applications at 35%, we know that many companies are still relying on paper-based initiatives to manage their workplace safety programs. If you’ve embraced one of these software solutions, then you’ve already automated data capture and completed the first step towards improving EHS program performance.
The next phase is to then extend data capture to a mobile device. Mobile applications enable automated workflows and completion of inspection reports, audits and questionnaires – providing immediate visibility into any task at any given time. Data can be collected from any workplace (online and offline). Mobile functionality puts your safety initiatives in each employee’s hand - empowering your workforce and entrenching their participation in your programs.
Data Quality
The quality of the data input into a predictive algorithm will directly impact the accuracy of that algorithm. Today’s safety professionals are telling us it’s challenging to execute on the information at hand. There’s a great volume of siloed, inconsistent and incomplete data that needs to be cleansed before even basic reporting can be done. When it comes to preparing your organization for predictive analytics, take the time now to review and make sure your data is a priority. This means assessing the data on four criteria:
- Completeness
- Accuracy
- Consistency
- Timeliness
Completeness ensures the optimal operation of the predictive model. Accuracy ensures that the data being input into the model is without errors, typos, and is still valid. Consistency in data is important as it ensures related records are telling the same story. Timeliness accounts for the lag between the actual event and its recording. The timelier the data, the more accurate the algorithm and prediction.
Combining Human-Captured and Machine-Generated Data in Predictive Analytics
As part of our work in this field, we’ve spoken to many safety professionals and the consensus is that resource prioritization is still a struggle. They’re unsure which investments will have the biggest impact and lack foresight to identify risk before it happens. Where do the tangible results lie? Our initial findings include significant reductions in lost work days, decreased incident rates and improved contractor safety compliance.
While the value of employee participation in your EHSQ program cannot be understated – poor occupational health and safety practices are estimated at 4% of global GDP each year – safety professionals can’t rely on just observational data and gut instinct to prevent workplace incidents. The real value comes from incorporating front-line employee-generated data with machine-generated data. For example, the combination of human-generated data (such as training completed, behavioral-based inputs, observations, length of service, and incident investigations) with machine-generated data (such as fatigue levels, weather data, and slip detection in footwear) can provide a much more accurate prediction of the propensity of future accidents. Based on these predictions, the safety manager will be able to implement new training initiatives, shift changes and process improvements to mitigate future risk. The role of the EHS lead then becomes more about fostering and championing a strong safety culture across the organization.
Predictive Analytics for the Safety Professional
When it comes to implementing predictive analytics at your organization, it’s beneficial to look for a third party to assist you – especially one that has access to a vast amount of EHSQ data from across their client base. The more data within the system, the more data points and subsets available for the modeling of safety algorithms. Leading vendors will prioritize data privacy and confidentiality. A common means of instilling data privacy is through Differential Privacy. Differential Privacy adds a “noise” filter to the data which scrambles any personally identifiable information before it’s added to the algorithm. Differential Privacy is currently being used by leading organizations such as Apple, Netflix, and Uber. This will expand and extend the potential reach and impact of your safety initiatives. In addition, it will afford you the benefit of not having to compete for these limited corporate-wide resources including access to Data Scientists.
As you look to further reduce the impact and frequency of workplace accidents, continue to examine predictive solutions that can provide you with the foresight of where to look and what to action first to proactively improve your program performance. Predictive analytics will drive tangible results as well as directional advice. Leveraging technologies such as wearables and mobile apps provide a holistic view, combining a dual human and machine-generated data strategy. As a result, they enable and empower every employee to have a vested interest in their own contribution to building a safer workplace.